An Introduction to the Data envelopment Analysis (DEA)
DEA representing data envelopment analysis is considered as a powerful method employed in multi-criteria decision making that assesses the relative effectiveness of a set of decision-making units (DMUs). It is a non-parametric approach that assesses the effectiveness of DMUs based on their inputs and outputs. DEA is commonly employed in the different fields, such as finance, economics, management, and engineering.
The DEA assumes that multiple inputs and outputs are observed for each DMU, and also the effectiveness of the DMU is assessed through comparing the ratio of output to input with that of other DMUs. It can manage situations where several inputs and outputs are observed, and also no clear method to elucidate a single measure of the effectiveness is found.
The DEA provides many benefits over other approach. It needs no assumptions about the production function, and also the DEA can manage situations where several inputs and outputs are observed. Moreover, DEA can be applied to recognize the sources of ineffectiveness and provide methods to increase the performance of the DMUs.
In short, DEA can be considered as a very useful tool for decision-makers who want to assess the relative effectiveness of a set of DMUs having several inputs and outputs. Due to its flexibility, simplicity, and capability to present actionable insights, DEA can be considered as a popular method which can be used by scholars and professionals.
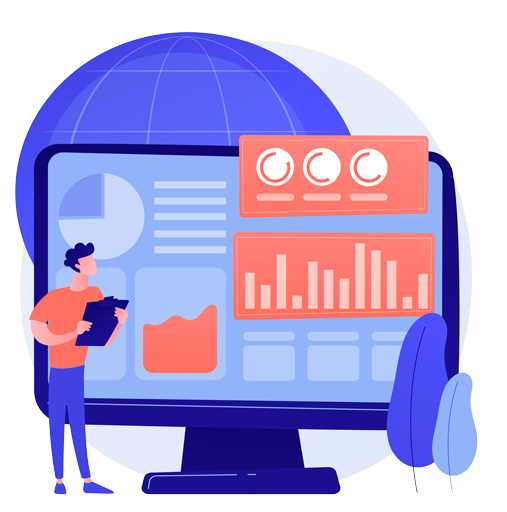
history of DEA
In1978, the masterminds, including Abraham Charnes, William Cooper, and Edwardo Rhodes collectively introduced a revolutionary concept called Data Envelopment Analysis (DEA). This method, rocking the foundations of the analysis of traditional decision-making unit (DMU), was particularly crafted for evaluating the effectiveness of DMUs having several inputs and outputs in a non-parametric fashion. As it is originally conceived for the realm of operational research, the comprehensive ability of DEA has transcended from its humble beginnings to become the best method for choice in the areas of finance, economics, and engineering. Since its inception until today, DEA has undergone large amount of refinements and modifications that have culminated in its radically distinctive and without equal ability to address challenges in the ever-changing field of decision-making. Its ability as a tool to detect the opportunities for improvement, and accurately measure the effectiveness of DMUs, has turned out to be such a stalwart in the arsenal of any organization seeking to achieve the optimum performance.
DEA method steps
Step 1: Defining the DMUs and their inputs and outputs
The first step is to detect the DMUs being assessed and the inputs and outputs related to each DMU. These inputs and outputs can differ according to the problem being analyzed, but it is important to define them accurately.
Step 2: Normalizing the data
When both the inputs and outputs are defined, the data should be normalized to guarantee that each DMU is assessed on the same scale. Several methods, such as the standardization and scaling are found to normalize the data, but it is important to choose the best method for the given problem.
Step 3: Determining the DEA model and solving it
The next step is to determine the DEA model and solve it so as to estimate the efficiency scores for each DMU. The DEA model is employed to calculate the relative effectiveness of each DMU with respect to its inputs and outputs as compared with other DMUs.
Step 4: Identifying the relative effectiveness of each DMU
When the DEA model is solved, the relative effectiveness of each DMU can be determined. DMUs with an efficiency score equaling to 1 are considered to be completely effective, while those with a score lower than 1 are not effective.
Step 5: Identifying the sources of ineffectiveness and suggesting the methods for improvement
The final step is to identify the sources of ineffectiveness and suggest the methods to improve the performance of the ineffective DMUs. This information can be applied to optimize the performance related to the DMUs and obtain better results.
In summary, DEA as a powerful method involves defining the DMUs and their inputs and outputs, normalizing the data, determining the DEA model, identifying the relative effectiveness of DMUs, and detecting opportunities for the improvement.
DEA method example
Here is an example of how the DEA can be used:
Suppose you are assessing the effectiveness of three banks, namely A, B, and C, based on two inputs, capital and personnel, and two outputs, loans and profits. The following data are considered for the banks:
Bank | Capital (millions) | Employees Loans (millions) | Capital (millions) | Profits (millions) |
A | 50 | 100 | 200 | 20 |
B | 70 | 150 | 250 | 25 |
C | 40 | 80 | 150 | 15 |
Step 1: Defining the DMUs and their inputs and outputs
Banks, including A, B, and C, are considered as the DMUs. Capital and Personnel are regarded as the inputs, and also loans and profits are considered as the outputs.
Step 2: Normalizing the data
The data can be normalized by a statistical technique called min-maxing, where each amount is divided by the highest amount for that input or output.
Bank | Capital (millions) | Employees Loans (millions) | Capital (millions) | Profits (millions) |
A | 0.714 | 0.667 | 0.8 | 0.8 |
B | 1 | 1 | 1 | 1 |
C | 0.571 | 0.533 | 0.6 | 0.6 |
Step 3: Determining the DEA model and solving it
Several methods are proposed to models related to DEA, but we will employ the basic input-oriented CCR representing Charnes-Cooper-Rhodes model. The objective of CCR model is to maximize the weighted sum of the outputs when keeping the inputs constant. The following results are obtained by using the DEA Solver Pro software:
Bank | Efficiency score |
A | 0.933 |
B | 1 |
C | 0.75 |
Step 4: Identifying the relative effectiveness of DMUs
Bank B with a score equaling to 1 is completely effective, while banks A and C receiving scores lower than 1 are not effective.
Step 5: Identifying the sources of ineffectiveness and suggesting the methods for improvement
Bank, including A and C can determine the sources of their ineffectiveness by comparing their inputs and outputs with those of the effective bank, B. They can then suggest the methods for improvement, such as an increase in their capital or their loan or profit management practices in order to enhance their efficiency score.
In conclusion, the DEA can be employed to assess the effectiveness of each DMU and detect the opportunities for the improvement. In this example, the DEA determined both the efficient and inefficient banks and provided a basis for suggesting the methods for improvement so as to enhance the effectiveness of banks which are ineffective.
Returns to scale in DEA:
Constant Returns to Scale (CRS):
CRS is observed when a proportional increase in all inputs and outputs leads to a proportional increase in the efficiency score of each DMU. In other words, as a DMU doubles its inputs and outputs, there will a twofold increase in its efficiency score. A CRS situation is ideal because it means that the DMU can effectively scale up its operations without showing a decrease in the effectiveness.
Increasing Returns to Scale (IRS):
IRS is observed when a proportional increase in all inputs and outputs is higher than that in the efficiency score of each DMU. In other words, as a DMU doubles its inputs and outputs, its efficiency score will be higher than double. An IRS situation implies that as the DMU scales up its operations; it becomes ever more effective, which can result in economies of scale.
Decreasing Returns to Scale (DRS):
DRS is observed when a proportional increase in all inputs and outputs is lower than that in the efficiency score of each DMU. In other words, if a DMU doubles its inputs and outputs, its efficiency score will be lower than double. A DRS situation implies that as the DMU scales up its operations, it becomes less effective, which can result in diseconomies of scale.
In general, the type of DRS is an important consideration in DEA because it can influence the efficiency scores and offer the need for changes observed in the scale of operations. DMUs with CRS can scale up their operations without influencing their effectiveness, while those with IRS may be able to receive an advantage from economies of scale. Also, DMUs with DRS may need to decrease their scale in order to maintain or increase their effectiveness.
DEA formula
The formula for DEA is obtained from a linear programming model comparing the inputs and outputs of each Decision Making Unit (DMU) with those of other DMUs to investigate the relative effectiveness of each DMU.
The basic DEA formula for the input-oriented model is as follows:
Maximize θ
subject to:
∑ᵢᵏ uᵢ xᵢ ≤ θ ∑ᵢᵏ uᵢ xᵢ⁰
∑ⱼⁿ vⱼ yⱼ ≤ 1
where
θ denotes the efficiency score of the DMU being assessed.
uᵢ represents the weight of the ith input.
xᵢ denotes the values of the ith input utilized by the DMU being assessed.
xᵢ⁰ represents the value of the ith input employed by the most effective DMU.
vⱼ denotes the weight of the jth output.
yⱼ represents the value of the jth output obtained from the DMU being assessed.
k denotes the number of inputs.
n represents the number of outputs
The first constraint guarantees that the DMU being assessed utilizes its inputs effectively compared to the most effective DMU, while the second constraint ensures that the DMU produces its outputs effectively in comparison with the other DMUs.
The output-oriented model bears a resemblance to the input-oriented model, but the functions of both inputs and outputs are contrary.
DEA application
DEA produces a diverse range of applications in the different areas, including
The assessment of performance: DEA can be employed to assess the performance of DMUs , such as firms , hospitals, schools, and other organizations with respect to their inputs and outputs.
Benchmarking: DEA can assist organizations to identify people who outperform their peers in their industry and learn their best practices.
The improvement of effectiveness: DEA can assist organizations to detect areas of ineffectiveness and recommend changes to their operations to improve the effectiveness.
The assignment of resources: DEA can assist organizations to assign resources more effectively by determining the optimal mix of inputs needed to obtain a given level of outputs.
Mergers and acquisitions: DEA can be employed to assess the potential synergies between two firms, presenting a more objective and data-driven method to the due diligence process.
The evaluation of the environmental consequences: DEA can be applied to evaluate the environmental effectiveness of various DMUs, including industries and cities, by determining their inputs and outputs associated with factors contributing to environment.
The management of the supply chain: DEA can be utilized to assess the suppliers’ performance and optimize the supply chain by determining the most effective suppliers and the optimal mix of inputs needed to obtain a given level of outputs.
In general, DEA can be used for many different purposes and can be applied in different contexts where the assessment of performance, benchmarking, and The improvement of effectiveness are favorable.
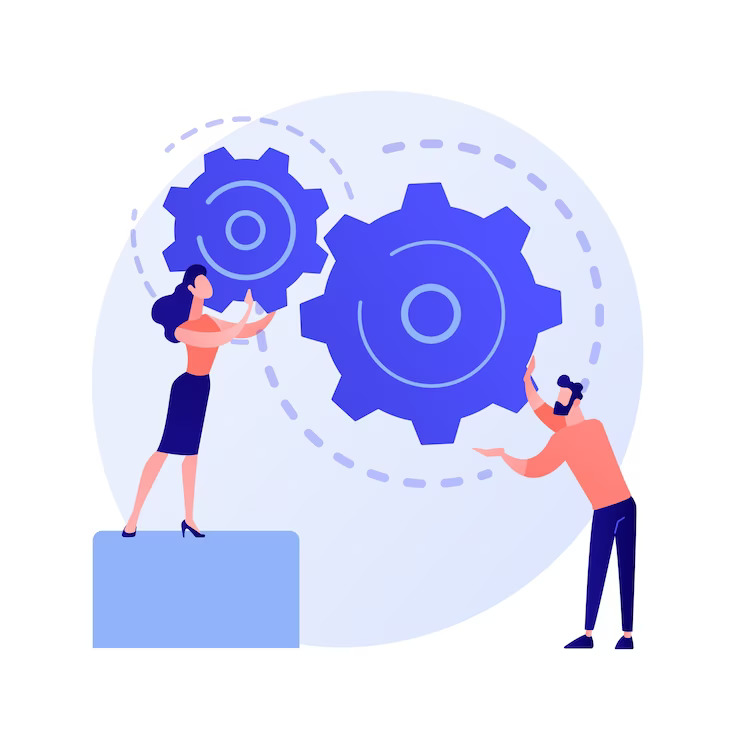
DEA papers
There are 10 studies conducted on Data Envelopment Analysis (DEA) published in 2021 and are presented in the following:
“A novel self-tuning DEA-based model for optimal forecasting of crude oil prices” by A. Daneshkhah and M. Makui
“Data envelopment analysis with non-convex production possibility sets” by S. Li et al.
“Exploring the efficiency of higher education institutions using an integrated DEA-Malmquist analysis” by N. Asgari et al.
“Assessing the efficiency and productivity of thermal power plants in India using DEA and Malmquist indices” by N. Bhattacharyya et al.
“A data envelopment analysis-based approach to evaluate and optimize the energy efficiency of urban buildings” by W. Jiang et al.
“An improved DEA-based model for selecting sustainable suppliers in the fashion industry” by K. Tseng et al.
“Evaluation of the financial performance of Indian public sector banks using DEA and Malmquist indices” by P. Sarkar et al.
“A new DEA-based framework for measuring corporate social responsibility performance” by A. Alimardani et al.
“Efficiency measurement of medical tourism in Asian countries using DEA and Malmquist indices” by T. Jindapon et al.
“A data envelopment analysis approach for measuring the efficiency of hospitals in the era of COVID-19 pandemic: Evidence from Turkey” by S. Ozcan and I. Kutlukaya.
The implementation of DEA models in Excel and MATLAB
DEA models can be implemented in Excel or MATLAB. Here’s how you can implement DEA models using these two software tools:
Excel:
Step 1: Import your data into an Excel spreadsheet. Put in order your inputs and outputs in separate columns.
Step 2: Compute the normalized data for each input and output variable.
Step 3: Utilize Excel’s Solver add-in to solve the linear programming problem for each DMU.
Step 4: Determine the efficiency score for DMUs based on the linear programming solution.
MATLAB:
Step 1: Import your data into MATLAB.
Step 2: Define the input and output matrices.
Step 3: Employ the DEA function in MATLAB’s Optimization Toolbox to solve the linear programming problem for DMUs.
Step 4: Determine the efficiency score for DMUs based on the linear programming solution.
There are advantages and disadvantages in using both Excel and MATLAB as they are implemented in DEA models. Excel is now widely available worldwide, but it may t be inappropriate for big datasets or complex models. MATLAB is more powerful
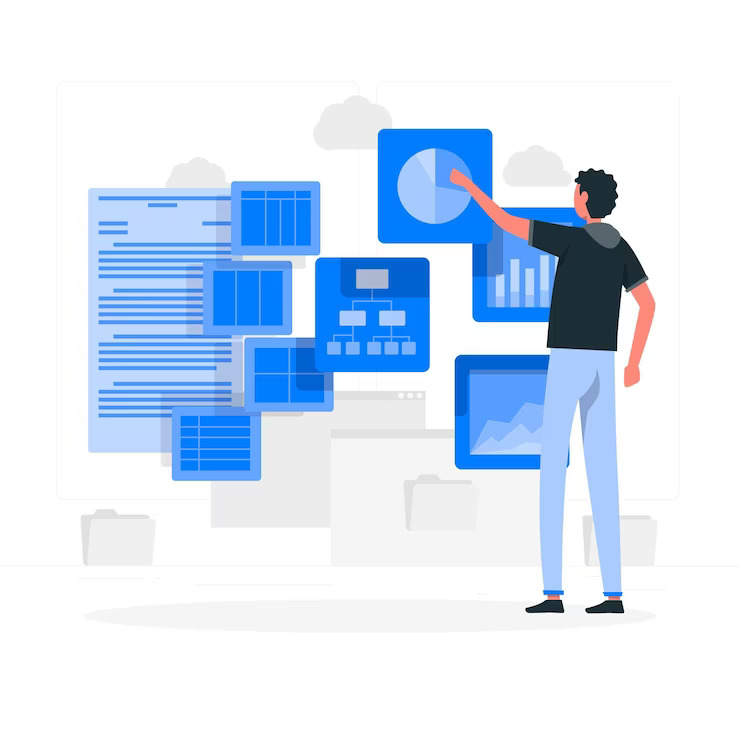
Limitations of DEA implementation in Excel
While Excel as a powerful tool is employed to analyze the data, some limitations to implementing DEA models in Excel are identified. Some of these limitations are as follows: The size of data: Excel puts a limit on the number of rows and columns that can be employed in a spreadsheet. This suggests that big data sets may not be managed effectively in Excel, particularly when determining the efficiency scores for DMUs.
Complexity of model: Excel has limited capabilities to solve complex optimization problems. DEA models can become considerably complex when dealing with several inputs and outputs, and also the use of Excel for solving such models can be time-consuming and error-prone.
The normalization of inputs and outputs: The normalization of inputs and outputs is considered as one of the main steps for DEA. This involves dividing each input and output by a reference amount. Excel needs further formulas to compute the normalized values, and this process can be error-prone and time-consuming.
Solver add-in: Excel’s Solver add-in is utilized to solve the linear programming problem for each DMU in DEA. However, Solver can sometimes present incorrect or infeasible solutions, particularly for complex models, resulting in the inexact DEA results.
Lack of advanced DEA features: Excel lacks some advanced DEA features, such as the capability to manage variable returns to scale (VRS) and non-discretionary inputs and outputs
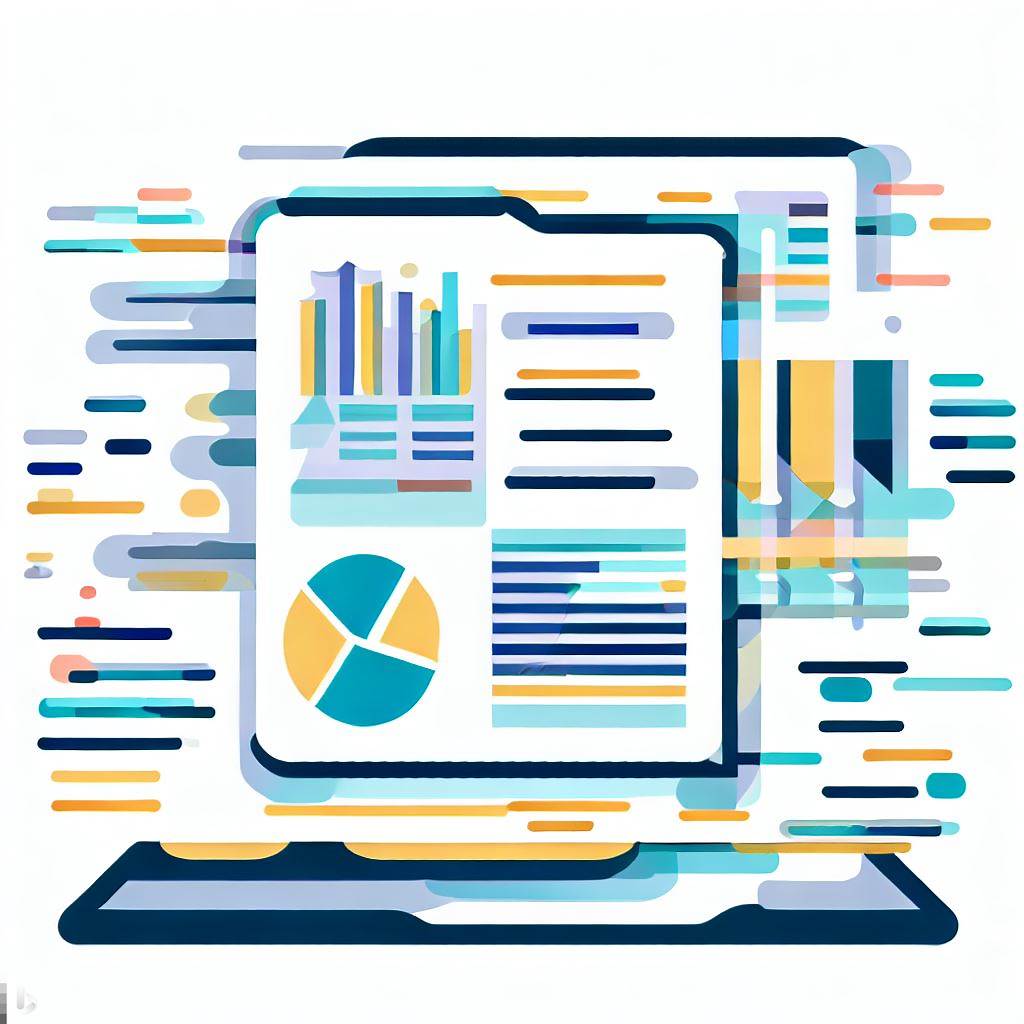
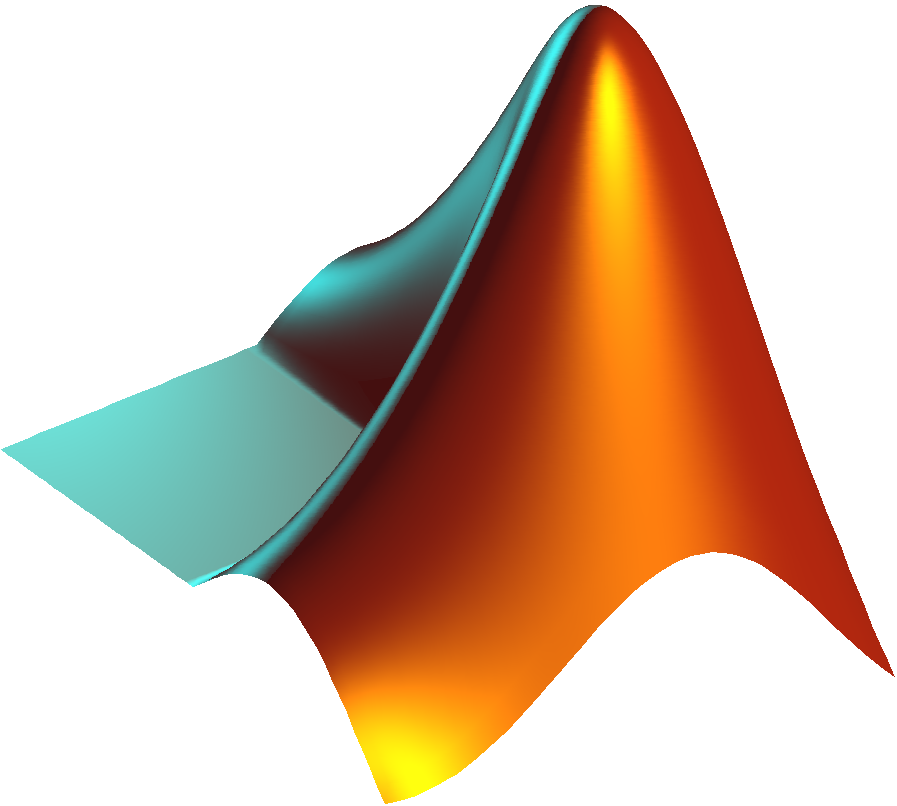
Limitations of DEA implementation in MATLAB
While MATLAB as a powerful tool is employed to implement the DEA, some limitations to its implementation are identified. Some of these limitations are as follows:
Learning curve: MATLAB as a programming language needs some programming knowledge. This implies that learning curve of MATLAB may be steeper than that of Excel or other DEA software.
Time-consuming: the optimization functions of MATLAB can be slow when dealing with big data sets or complex models. This can make the implementation of DEA models time-consuming, particularly when solving models with an enormous number of DMUs.
Cost: MATLAB as a proprietary software needs a license to use. This can make it a costly option, particularly for small businesses or scholars who have limited budgets.
Lack of user-friendliness: Unlike DEA, MATLAB is not definitely developed for the analysis of the DEA. This suggests that users may have to write code from scratch or use third-party toolboxes to implement DEA models, which can be more time-consuming and less user-friendly.
Lack of visualization tools: limited capabilities are identified for MATLAB when visualizing the results of DEA. Users may have to export the findings to other software tools for creating data visualizations, which can be time-consuming.
DEA onlie software
Some benefits of using online DEA software are as follows:
Accessibility: Online DEA software is available from anywhere with an internet connection, allowing the users to easily share their data and collaborate with others. This can be particularly useful for team members who work remotely or live in different locations.
User-friendly: Online DEA software, which is usually designed with a user-friendly interface, needs the minimum programming knowledge. This indicates that users can rapidly learn to apply the software and start analyzing their data.
Scalability: Online DEA software can easily manage big data sets and complex models without needing further hardware or software upgrades. This makes it a scalable solution that can grow with needs of the users.
Automatic updates: Online DEA software often have automatic updates, demonstrating that users always have access to the most recent features and functionality.
Real-time support: Most of people who provide online DEA software offer real-time support via chat or email, which can assist users to rapidly solve any problems faced by them while employing the software.
Cost-effective: Online DEA software is often provided on a subscription basis, which can be more cost-effective than purchasing software outright. This also removes the need for the costly hardware and software upgrades.
Generally, online DEA software offers may benefits over conventional software, making it an attractive option for businesses and scholars who look for streamlining their DEA analysis.